- What is AI-Based Sentiment Analysis?
- Approaches to Sentiment Analysis: Understanding the Methods
- Rule-Based Approach
- Machine Learning (ML) Approach
- Hybrid Approach
- 10 Benefits and AI Sentiment Analysis Use Cases
- Reputation Management
- Product Analysis
- Voice of Customer Analysis
- Market Research Competitor Analysis
- Customer Support-Feedback Analysis
- Real-Time Customer Insights
- Product Development Optimization
- Personalized Marketing Campaigns
- Predictive Analytics
- Risk Mitigation
- Conclusion
- Faq’s
Understanding and connecting with customers’ thoughts and emotions has become essential in this customer-centric world. Studies reveal that a remarkable 95% of consumers usually check online reviews before making purchasing decisions. Interestingly, 58% of these individuals are even willing to invest more money in products endorsed by positively reviewed brands.
86% of consumers are willing to pay more for a good customer experience. According to this data, the buying process, which includes initial contact and post-sales assistance, has a greater impact on buying decisions than the product itself.
This indicates that customer opinions hold great importance. This is where AI sentiment analysis plays a crucial role. It functions as an intelligent tool that assists businesses in understanding their customer’s thoughts and emotions. AI-based sentiment analysis also helps businesses to identify customer trends, allowing them to make informed decisions. Additionally, it helps businesses to develop better customer service strategies, which can lead to increased customer satisfaction and loyalty.
This article explores ten benefits of AI for sentiment analysis for businesses along with real-life examples of how it is transforming the way companies operate and enhance customer satisfaction.
What is AI-Based Sentiment Analysis?
AI sentiment analysis, also known as sentiment analysis or opinion mining, is an advanced technology that automates the examination of text data to determine the expressed sentiment or emotional tone. This technology utilizes artificial intelligence (AI) and natural language processing (NLP) techniques to analyze and comprehend emotions, opinions, and subjective information conveyed through written or spoken language.
NLP enables computers to comprehend and interpret human language, encompassing nuances, context, and sentiment.
Meanwhile, machine learning algorithms play an integral role in NLP sentiment analysis by being trained on extensive datasets with labeled sentiments. This training allows them to identify patterns and associations between words, phrases, and sentiments. Consequently, these empowered algorithms can automatically classify new text data based on sentiment.
AI algorithms analyze and interpret human sentiments through a well-defined process. First, the text data goes through preprocessing to eliminate irrelevant information, allowing the algorithm to focus on meaningful content. Next, relevant features are extracted from the text and transformed into numerical representations that machine learning models can process in a step called feature extraction. These models are trained using labeled data, which includes text samples with known sentiment labels. This training enables them to predict sentiment in new, unlabeled text data.
AI sentiment analysis is a versatile technology that can be applied to analyze various types of data, including written text, social media content, product reviews, and customer feedback. Its primary purpose revolves around assessing the sentiment expressed across different platforms and domains. For instance, it is commonly utilized to discover public sentiment on social media platforms, monitor customer satisfaction through product reviews, and evaluate sentiment analysis in customer feedback. These sentiment analysis AI applications offer valuable insights for businesses and individuals alike, enabling them to better understand and respond to human sentiment.
Approaches to Sentiment Analysis: Understanding the Methods
Sentiment analysis leverages various techniques to determine the emotional tone behind a text. Here are the three primary approaches used in sentiment analysis software:
Rule-Based Approach
The rule-based approach relies on predefined lists of words (lexicons) with assigned sentiment scores. The system scans the text for these words and calculates an overall sentiment score based on the presence and weight of the words found.
Example: “The food was good, but service was poor” scores positive for “good” and negative for “poor,” giving an overall mixed sentiment.
Machine Learning (ML) Approach
The ML approach involves training algorithms on large datasets of text that have been labeled with sentiment tags. The model learns to recognize patterns and predict the sentiment of new, unlabeled text based on these patterns.
Example: An ML model identifies “great movie” as positive and “boring plot” as negative, predicting the sentiment of “great movie but boring plot” as mixed.
Hybrid Approach
The hybrid approach combines rule-based and ML methods to leverage each of their strengths. It aims to enhance both speed and accuracy by integrating predefined rules with machine learning insights. This method can offer more nuanced sentiment detection and adapt to varying contexts more effectively.
Example: A hybrid system recognizes “not bad” with rules and uses ML to confirm it’s positive in context, interpreting “not bad but not great” as slightly positive.
10 Benefits and AI Sentiment Analysis Use Cases
AI for sentiment analysis provides actionable insights into customer emotions and opinions, aiding decision-making processes. Here are the 10 benefits of AI sentiment analysis along with use cases examples of the same:
Reputation Management
One of the most crucial AI sentiment analysis benefits for any business is understanding the public perception of its brand. This holds great importance for tech companies, clothing brands, and virtually every type of business possible. This is where AI for sentiment analysis becomes valuable. Let’s know into how it operates:
- Brand Feelings Surveillance: By employing AI-based sentiment analysis, companies can closely monitor the public perception of their brand. This entails paying attention not only to the positive comments but also to any negative or even neutral feedback.
- Noticing Specific Feeling: Sentiment analysis enables the identification of specific emotions expressed by customers. It assists in understanding a range of feelings, including happiness, frustration, and everything in between. This analysis provides valuable insights into customer sentiment.
- Finding Patterns and Trends: By analyzing a large amount of customer feedback, sentiment analysis can identify recurring patterns and trends. It’s like identifying popular topics of conversation among numerous individuals.
- Paying attention to influential individuals: These individuals hold significant sway over public opinion. By employing sentiment analysis, businesses can identify these key figures and collaborate with them to enhance their brand’s reputation and standing.
Let’s see how KFC did it:
KFC is a prime example of how AI sentiment analysis can be utilized to enhance a brand. While other restaurants have attempted to promote healthier options and positivity, KFC took a different approach. Recognizing the strength of their existing reputation, they opted for an alternative strategy instead of implementing drastic changes. Their tactic? Embracing the playful side and embarking on a joyful journey without compromising their established identity.
KFC incorporated elements from popular culture, such as well-known symbols and internet jokes, to promote their food. This approach reignited people’s interest in KFC. By utilizing sentiment analysis, they were able to evaluate the effectiveness of their strategies and make improvements to enhance their brand further.
Product Analysis
AI-based sentiment analysis expands beyond brands; it also provides valuable insights into understanding products. It can be an advanced tool, functioning like an attentive listener capturing people’s opinions on various aspects of a product, its performance, unique features, and user experience.
In the early stages of developing a new product, companies need to prioritize its importance. Properly testing the product with real users becomes essential to ensure its quality. This is where AI for sentiment analysis plays a significant role. It helps companies measure user feedback and make necessary improvements.
At this stage, AI sentiment analysis does some important jobs:
- Collecting Feedback: This process involves gathering all the comments and opinions expressed by people regarding the product.
- Sorting Feedback: This lets you see what people like and dislike about the application.
- Understanding What’s Important: This tool determines the key aspects of feedback, such as highlighting discussions on product speed or user-friendliness.
- Learning from others: This involves analyzing feedback and opinions about similar products offered by other companies to enhance their product. Such insights help them make improvements that align with customer needs and preferences while ensuring a better overall offering.
Now, let’s see how Google uses this:
Google diligently pays attention to user feedback. Instead of examining individual comments, they thoroughly analyze the broader perspective. Does the public opinion on Chrome lean towards positive or negative? They assess whether the majority of people have favorable or unfavorable things to say about Chrome. Specifically, the focus lies on gathering feedback about various aspects of Chrome. This includes its performance, customizable options, security features, and visual aesthetics.
Voice of Customer Analysis
To ensure the success of any business, it becomes crucial to have a thorough understanding of your customers’ preferences and needs. It’s like aiming at a target, you must precisely identify its location and utilize the appropriate tools for hitting it accurately.
However, there is a challenging aspect to consider: the thoughts and opinions of your customers are spread across different platforms, making them difficult to consolidate. Sometimes, their perspectives on your product can be quite diverse. This is where customer sentiment analysis becomes valuable. It helps in various aspects:
- Understanding Your Audience: Let’s take an example of a department store, where you desire to know the desires of diverse groups of shoppers. AI-based sentiment analysis comes to your help, examining various customer segments and determining their needs, expectations for similar products, and preferred choices.
- Customer Feedback Analysis: Consider a product, such as a hotel, and the desire to enhance it based on customer input. The sentiment analysis evaluates direct and indirect comments about the hotel. It’s like actively listening to customers as they express their experiences.
Now, let’s see how Tripadvisor uses this:
TripAdvisor, a platform dedicated to travel reviews, excels in utilizing AI based sentiment analysis to process the numbers of feedback received from travelers effectively. This method allows them to identify essential insights within customer reviews and enhance their services accordingly.
Market Research Competitor Analysis
Market research is a crucial aspect of understanding the business environment. Combining it with competitor analysis provides a comprehensive view of the market. Competitor analysis is a valuable AI sentiment analysis use cases. The process involves analyzing your brand as well as how your competitors are perceived. Sentiment analysis can provide useful insights into this context. Let’s explore how it helps:
- Gathering Data from Multiple Sources: Sentiment analysis is capable of collecting data from a variety of platforms. These include user-generated content such as comments and reviews, influencer content, news articles, and even the content produced by your competitors. This enables you to obtain a comprehensive view of the sentiment surrounding your brand or industry.
- Extracting Insights: In the process of analyzing this data, valuable insights can be gained regarding the overall market perception, specific aspects, and their reception, as well as consumer sentiments towards your competitors.
Now, let’s explore how a leading technology company like Apple leverages AI based sentiment analysis for conducting market research and analyzing competitors. By utilizing sentiment analysis, Apple gains valuable insights into customer perceptions and attitudes, allowing them to make informed decisions based on the feedback received.
By analyzing competitors and their market movements, Apple gains valuable insights. These insights encompass various aspects, such as their brand’s value proposition, problem-solving capabilities, introduction of new features, and important milestones.
Customer Support-Feedback Analysis
Customer support is a major area where sentiment analysis can have a significant impact. It involves effective understanding and response to customer feedback. Let’s know AI-driven sentiment analysis plays a crucial role in this context:
- Insight into Customer Perceptions: Sentiment analysis helps businesses measure customers’ perceptions of their products or services. This analysis discloses the overall impression customers have, whether positive or negative. Additionally, it provides deep analysis of specific product elements and can even evaluate service effectiveness. Detailed analyses may include aspects such as response time or the quality of interactions.
- Automation with Intent Analysis: It can automate routine queries. These include frequently asked questions or requests for information about product usage. By automating these processes, customer support becomes more streamlined, leading to quick and efficient responses.
- Customer Prioritization and Workflow Management: It involves the practice of prioritizing customers based on their sentiments. This approach ensures that dissatisfied customers are promptly attended to. For example, if a customer expresses dissatisfaction, their ticket can be given priority for faster resolution.
Now, let’s explore AI sentiment analysis examples where companies effectively utilize AI-driven sentiment analysis in their customer support processes.
T-Mobile employed sentiment analysis with AI to examine customer feedback and identify prevalent issues. By utilizing generative AI sentiment analysis, T-Mobile swiftly detected areas of concern and promptly addressed them. This proactive approach resulted in an impressive 73% reduction in customer complaints.
Ford utilizes advanced AI for sentiment analysis to carefully analyze customer feedback and identify emerging trends regarding vehicle performance and overall customer satisfaction. This real-time analysis enables Ford to promptly pinpoint any issues or areas for improvement in their vehicles and services, ensuring continuous enhancements are made.
Real-Time Customer Insights
AI-based sentiment analysis revolutionizes the way businesses gather real-time customer insights. The sentiment analysis in social media achieves this by actively monitoring social media platforms, review sites, and various online channels. This capability empowers companies to promptly obtain feedback and stay updated on the dynamic customer sentiments.
Let’s understand this with the Airbnb example:
Airbnb, a leading player in the global accommodation industry, utilizes generative AI sentiment analysis to closely monitor interactions between guests and hosts. This proactive approach involves analyzing the sentiments expressed within guest reviews and messages exchanged between users. By doing so, Airbnb can swiftly identify emerging issues and concerns in real-time.
For example, when multiple guests express dissatisfaction with a particular property’s cleanliness or report safety concerns through messages, Airbnb’s sentiment analysis algorithms quickly identify these sentiments. This early detection enables Airbnb to take immediate action by communicating with the host to address cleanliness issues or verifying safety protocols.
Product Development Optimization
AI-based sentiment analysis has revolutionized product development, providing businesses with invaluable insights into customer preferences and pain points. It serves as a guiding tool in the complexity of optimizing offerings by analyzing customer feedback and reviews. This enables companies to gain a deeper understanding of what truly resonates with their audience and identify areas that need improvement.
Let’s understand this with the Amazon example:
Amazon, the renowned global e-commerce giant, employs sentiment analysis to meticulously examine a vast collection of customer reviews. By leveraging this advanced analysis, Amazon gains invaluable insights that directly influence its product development strategies. For instance, when customers consistently praise a specific feature like exceptional durability or user-friendliness, sentiment analysis with AI brings this pattern to light.
In order to put customer insights into action, Amazon divides the process into several actionable steps. They prioritize enhancing products that align with customer preferences, ensuring that future iterations maintain or amplify these strengths. Additionally, Amazon strategically highlights these exceptional features in their marketing campaigns to forge a deeper connection with their target audience.
Amazon’s use of AI for sentiment analysis showcases how businesses can optimize product development by actively listening to customer feedback. This boosts satisfaction and also ensures the delivery of products that truly meet market demands.
Personalized Marketing Campaigns
AI-based sentiment analysis is essential in helping businesses develop highly personalized marketing campaigns. By understanding the sentiments of individual customers or customer segments, companies can customize their marketing messages and content to resonate deeply with their audience on a personal level.
Let’s understand with Spotify example:
Spotify is a widely used music streaming service that utilizes AI enabled sentiment analysis to curate personalized playlists and recommendations for its users. By examining user sentiments towards various music genres, artists, and songs, Spotify’s recommendation engine can construct playlists that cater to each user’s unique preferences. This high level of personalization keeps users engaged and content with the platform.
Predictive Analytics
AI-based sentiment analysis goes beyond understanding present sentiments. It also serves as a powerful tool for predictive analytics, benefiting businesses in various ways. By analyzing historical sentiment data, companies can predict future trends in customer sentiment and accordingly adapt their strategies. This enables them to make informed decisions when it comes to product launches, marketing campaigns, and other crucial aspects of their operations.
IBM includes sentiment analysis as part of its Watson suite of services. These services are widely used across industries such as finance and healthcare. Sentiment analysis helps businesses in these sectors predict market trends, understand customer behavior, and identify emerging risks. For example, sentiment analysis in finance or financial institutions utilize sentiment analysis to analyze trends related to economic indicators. This enables them to make informed investment decisions that optimize profitability while minimizing potential losses.
Risk Mitigation
Happy and satisfied employees have a higher likelihood of delivering exceptional customer experiences. Businesses can internally utilize sentiment analysis to assess employee satisfaction and pinpoint areas for workplace improvement. This, in turn, encourages a more motivated and productive workforce.
For example, UnitedHealth Group, is a renowned company using sentiment analysis in Healthcare. It employs AI enabled sentiment analysis to gauge employee sentiments through feedback surveys. This enables them to gain valuable insights into employee perspectives on workplace conditions, management, and benefits. Identification of areas where employees express dissatisfaction empowers UnitedHealth Group to proactively address these concerns and promote a happier and more engaged workforce. Ultimately, this positively impacts the quality of care provided to customers.
Conclusion
AI-based sentiment analysis enables businesses to gain a deeper understanding of their customers, enhance brand reputation, and optimize products/services. It offers real-time insights, identifies growing trends, and facilitates data-driven decision-making.
Looking to harness the power of AI-based sentiment analysis for your business? Consider partnering with Appinventiv. With our experience and cutting-edge technology and by being the top-most AI development company, we can help you leverage customer feedback and drive success. Take the next step with Appinventive today!
Faq’s
Q. How AI Sentiment Analysis Works?
A. Sentiment analysis in AI combines natural language processing and machine learning techniques. Its main objective is to evaluate the emotional tone or sentiment conveyed in textual data. By categorizing sentiments as positive, negative, or neutral, this process provides valuable insights into public opinion, customer feedback, and the emotional context of textual information.
Q. What role does machine learning play in sentiment analysis?
A. Machine learning plays a significant role in sentiment analysis. It is used to train models that can understand and categorize text based on sentiments. These models acquire knowledge from extensive labeled data, allowing them to identify language patterns, context, and subtle nuances. AI sentiment analysis models often utilize machine learning algorithms such as neural networks and support vector machines. Once developed, these models efficiently analyze and classify large amounts of textual information, making sentiment analysis highly effective in various applications ranging from customer feedback analysis to social media monitoring.
Q. What is Sentiment Analysis in AI?
A. AI sentiment analysis involves collecting text data and applying preprocessing techniques to clean and standardize the data. Subsequently, machine learning algorithms are utilized to categorize the sentiment of the text as positive, negative, or neutral. This process facilitates a thorough understanding of the expressed sentiment.
Q. What are some of the challenges associated with AI sentiment analysis, and solutions to tackle them?
A. Here are the common challenges associated with AI sentiment analysis, along with solutions:
1. Data Quality and Bias: Inaccurate sentiment analysis results can stem from poor-quality or biased data.
Solution: Employ balanced datasets and perform regular audits to detect and address biases.
2. Handling Nuances and Sarcasm: Sarcasm and nuanced language can lead to misinterpretation by AI models.
Solution: Use advanced contextual algorithms and diverse training data to better recognize subtle language cues.
3. Cultural and Linguistic Variability: Sentiment analysis models may struggle with different languages and cultural contexts.
Solution: Train on multilingual and culturally varied datasets to enhance model adaptability.
4. Idiomatic and Contextual Understanding: Models can be confused by idiomatic expressions and contextual nuances.
Solution: Integrate contextual embeddings and include idiomatic phrases in training data.
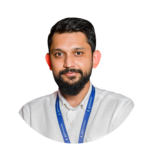
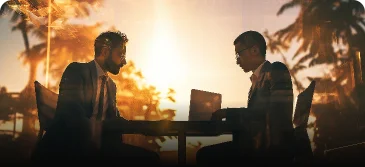
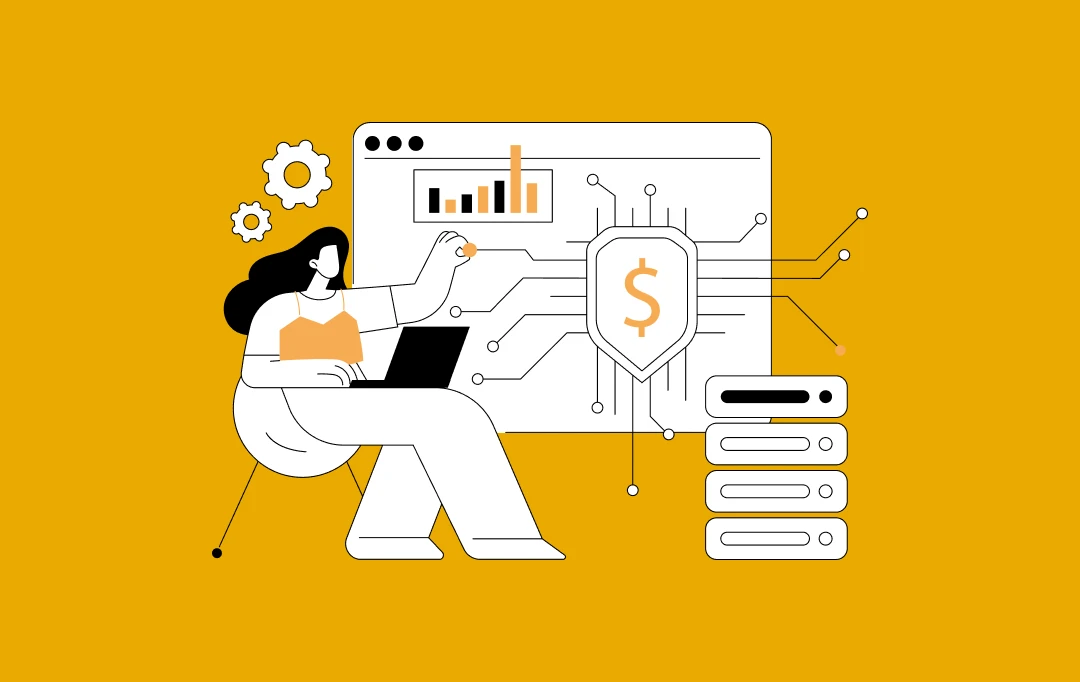
How Much Does it Cost to Build a Custom AI-based Accounting Software?
The accounting industry has been evolving very fast, and the expanding use of AI in accounting is becoming evident. According to The State of AI in Accounting Report 2024, 71% of accounting professionals believe that artificial intelligence in accounting is substantial. Considering the given number, it is easy to grasp why companies are keen on…
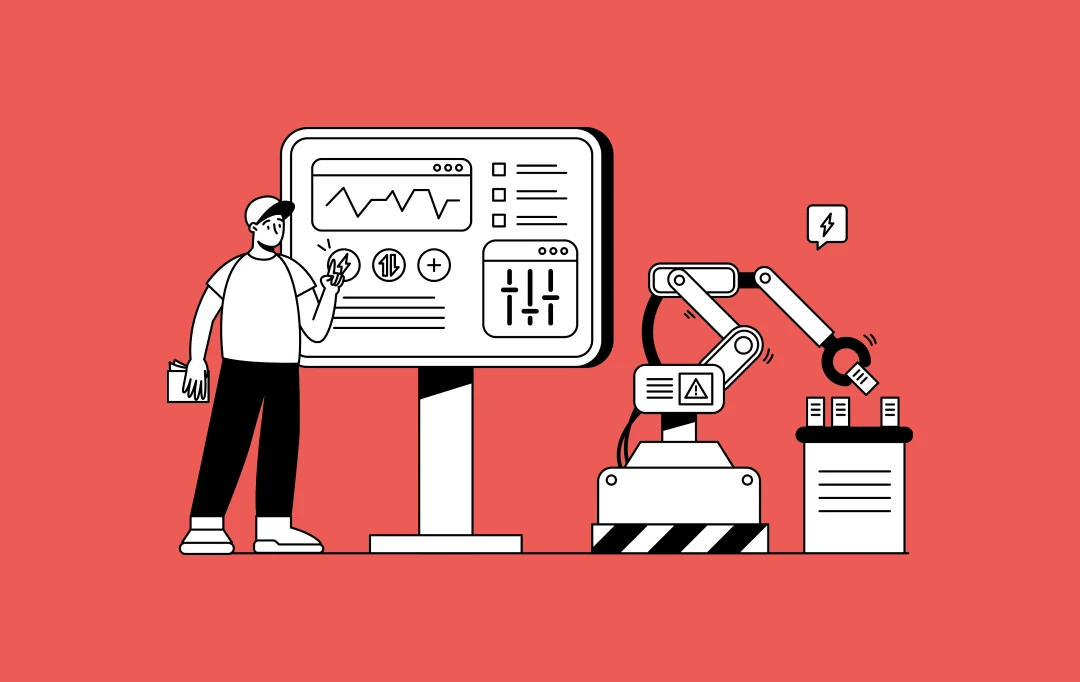
Generative AI in Manufacturing: 10 Popular Use-Cases
Introduction Manufacturing is truly getting a serious makeover for the future, and it's not full of buzzwords and techno-speak; with the dawning of AI technologies, manufacturing is no longer about nuts and bolts and conveyor belts. Yes! We are talking about how Generative AI in manufacturing is transforming the entire industry. It was the next…
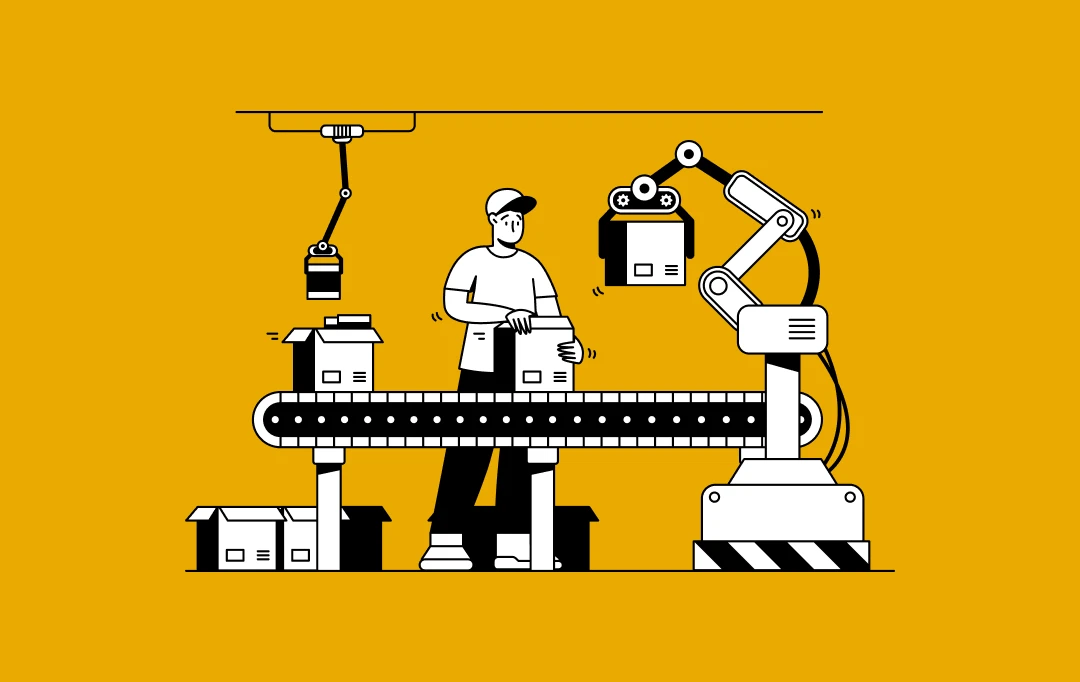