- Understanding the Manifold Benefits of Predictive Analytics in Healthcare
- Top Ten Use Cases of Using Predictive Analytics in Healthcare
- 1. Predictive Analytics Prevent Patient’s Readmission
- 2. Healthcare Predictive Analytics Enhance Cybersecurity
- 3. Managing Population Health
- 4. Streamlining the Submission of Insurance Claims
- 5. Analyzing Equipment Maintenance Requirements
- 6. Preventing Patient Deterioration in ICUs and General Hospitals
- 7. Suicide Attempt Prediction
- 8. Improving Patient Engagement
- 9. Minimizing Missed Appointments
- 10. Detecting Early Signs of Sepsis
- In Conclusion
- FAQ’s
Machine learning and artificial intelligence is a technological revolution that is touching every industry across the globe including healthcare. It changes the way doctors treat patients and prevent disease. Smart algorithms and thorough data analyses enable healthcare providers to identify possible health hazards. They would also help fine-tune treatment plans and optimize results in patients.
The global market of predictive analytics for healthcare is growing. However, it had a market value of $11.7 billion in 2022. Its CAGR is predicted to be approximately 24.4% over the period from 2023 to 2030. Such an unprecedented development has been necessitated by the urgent need to improve the outcomes and reduce the cost of delivering health care services.
Predictive analytics for healthcare is very important since people today want affordable, successful, and personalized treatment programs. By using this advanced approach, they help healthcare institutions to come up with tailored treatments and meet the growing demand efficiently. This article will examine 10 examples of predictive analytics in healthcare, which show how technology influences healthcare.
Understanding the Manifold Benefits of Predictive Analytics in Healthcare
Predictive analytics in healthcare is essentially using advanced data analysis on past health data. The goal is to find useful patterns and trends within this data, which can help healthcare professionals predict future health events and outcomes accurately. Using intricate algorithms and smart analytical methods, healthcare providers can spot possible health risks ahead of time, foresee the emergence of diseases, and predict how patients might react to different treatments.
Here are some of the most remarkable benefits of predictive analytics in healthcare:
- By thoroughly analyzing patient data, such as medical histories, diagnosis details, and treatment results, predictive analytics allows healthcare professionals to create intervention and treatment plans that specifically cater to each patient’s unique needs.
- Predictive analytics in healthcare also facilitates tailored approach that not only improves patient results but also boosts the effectiveness of healthcare delivery.
- Predictive analytics offers a practical way for healthcare providers to foresee possible health issues in chronic condition patients. This process allows for appropriate action to be taken promptly, preventing damaging consequences.
- It also lets hospitals and healthcare facilities manage their resources more efficiently, for instance, by predicting the number of patient admissions, ensuring optimal use of beds, and coordinating the distribution of staff and medical supplies in a timely manner.
- Furthermore, predictive analytics is essential in improving the accuracy of diagnoses. It helps detect diseases earlier and guides the creation of specific preventive strategies.
- Predictive analytics helps healthcare providers make decisions not just based on their expertise but also on actual data. It leads to better care for patients, smooth operations, and smarter use of resources.
These benefits of predictive analytics in healthcare contribute to making healthcare services the best they can be overall. As technology gets more sophisticated and our ability to analyze data improves, predictive analytics is set to take on a bigger role in shaping the future of healthcare.
Top Ten Use Cases of Using Predictive Analytics in Healthcare
Predictive analytics for healthcare is changing the healthcare sector in multiple ways. From improving healthcare outcomes to better allocation of resources, predictive analytics transforms the way patients receive healthcare. Here are ten predictive analytics in healthcare examples that offer the most value to healthcare providers:
1. Predictive Analytics Prevent Patient’s Readmission
Hospital readmissions are a cost issue that costs Medicare alone over two billion dollars annually. Readmissions have been highlighted by the Hospital Readmission Reduction program under Medicare, with 82% of participating hospitals penalized for increased readmission rates.
Predictive analytics for healthcare aids in the identification of at-risk patients to assist in implementing specialized follow-ups that can ensure appropriate discharge directives to forestall re-admissions.
A great example is UnityPoint Health, where predictive analytics models for healthcare assessed readmission risk scores for each patient. Using this tool well, A senior physician was able to predict and prevent a patient’s readmission within thirty days through the early treatment of the symptoms. Within 18 months after implementing predictive analytics, UnityPoint Health managed to reduce all-causes readmission by 40%.
These examples show the impact of predictive analytics in healthcare, containing medical care costs, improving healthcare outcomes, and reducing stress on healthcare resources.
Also read: The Ultimate Business Guide to Healthcare Application Development in 2023
2. Healthcare Predictive Analytics Enhance Cybersecurity
Cyberattacks on healthcare are a major issue, as evidenced by the Healthcare Data Breach Report from the HIPAA (2014). For instance, the report revealed that information was stolen prior to encryption in most of these ransomware attacks. In addition, April 2021 also reported 62 breaches in the healthcare industry, of which 7 compromised more than 100,000 records each.
As such, cybersecurity predictive analytics has increasingly become a viable solution for many healthcare organizations. These organizations will assess the transactional risks of online transactions using a model that is predictive and integrates it with artificial intelligence. For example, the system may allow a user to log in and provide multi-factor authentication or block high-risk processes. Moreover, predictive analytics models for healthcare allow for constant surveillance of data access and sharing, promptly picking up on any irregular tendencies indicating possible intrusions.
In the domain of cybersecurity, healthcare predictive analytics functions across two main categories, each encompassing various subtypes:
- Vulnerability-based solutions: Such weaknesses in the healthcare system are referred to as the Common Vulnerabilities and Exposures (CVE).
- Threat-focused platforms: These are meant to be ahead indicators of threats that may undermine security in the system.
3. Managing Population Health
Managing population health is a significant area where healthcare predictive analytics plays a crucial role, encompassing three key aspects:
Identifying Chronic Diseases
Through predictive analytics, healthcare institutions can identify and treat people before they develop chronic conditions. It is, therefore, an analytical approach that scores patients on some characteristics, including demographics, disabilities, age, etc., and their past care patterns.
Identifying Disease Outbreaks.
Predictive analytics has shown its strength in diagnosing disease outbreaks such as COVID-19. BlueDot is a Canadian company that used predictive analytics to issue an alert about unusual pneumonia cases in Wuhan on December 30, 2019, earlier than the formal announcement by WHO of COVID-19. In addition, the University of Texas Health Science Center at Houston (UTHealth) designed a predictive analytics tool for COVID-19 tracking, featuring a complete public health dashboard that displays current and projected pandemic spread trends.
4. Streamlining the Submission of Insurance Claims
Another area where predictive analytics can prove greatly beneficial in healthcare is in speeding up insurance claims submissions. With the help of these tools, hospitals can not only speed up the insurance claims process but also cut down on mistakes.
5. Analyzing Equipment Maintenance Requirements
While the earlier examples mainly highlighted how predictive analytics is used in clinical settings, it’s important to note that its benefits in healthcare also stretch to improving operations.
Predictive analytics has been used in many fields, for instance, in aviation, where it helps anticipate maintenance needs before they cause issues. By studying data from different parts of an airplane, technicians can replace mechanical parts before they fail. Similarly, healthcare operations can profit from this kind of predictive strategy, too.
Consider this: certain parts of medical machinery, like MRI scanners, gradually wear down over time because of regular usage. If health organizations can reliably predict when these parts may need to be replaced, hospitals can plan and schedule maintenance during times when they’re least busy. This way, possible disruptions to both healthcare providers and patients are kept to an absolute minimum.
Predictive analytics help streamline the process by making it possible to actively monitor and analyze technical data from MRI scanner sensors remotely. This enables us to detect possible technical problems early on, offering a chance to address them promptly through replacement or repair. In the future, hospitals could imagine a situation where each medical device and piece of equipment has its detailed digital twin that’s continuously updated with current data. This will help forecast future usage and maintenance requirements.
6. Preventing Patient Deterioration in ICUs and General Hospitals
In both intensive care units (ICUs) and general hospital wards, it’s imperative that doctors and nurses quickly detect any decline in a patient’s health. This is especially true when immediate action can mean the difference between life and death. This was a concern even before the COVID-19 pandemic. ICUs in several countries, including our own, were already stretched thin due to a growing older population, intricate surgical operations, and a lack of enough intensive care experts. Now, with the pandemic worsening the situation, the healthcare field desperately needs technological support to make quick, informed decisions.
Keeping a constant eye on a patient’s vitals can help predictive software identify those likely to require help within the next hour. This allows caregivers to step in at the earliest signs of declining health. The role of predictive analytics in healthcare in gauging the risk of a patient passing away or needing to be readmitted within two days after leaving the ICU. This knowledge helps caregivers make informed decisions regarding patient discharge.
Predictive algorithms are now being used in settings like tele-ICUs. Here, constant monitoring is carried out by doctors specialized in intensive care and critical care nurses who aren’t in the same location as the patient.
This enables them to step in when needed swiftly. Moreover, predictive analytics help spot the earliest signs of patients starting to do poorly in general wards, places where such signs could go unnoticed for quite some time. Automated systems for early warnings quickly get Rapid Response Teams to react, which has resulted in a meaningful drop in negative incidents by 35% and heart attacks in hospitals by 86%, according to a report from Philips.
Wearable biosensors, which can be subtly attached to a patient’s chest, have greatly improved the ability of healthcare providers to identify early indications of patient decline. They are particularly helpful for patients who move through various care settings within the hospital.
These biosensors continuously gather and send critical health information like heartbeat and breathing rates. They also monitor contextual factors like body posture and the patient’s activity levels. The advantage of these devices is that they allow for remote monitoring, reducing the need for recurring in-person health checks. This has been particularly beneficial in treating patients with COVID-19.
7. Suicide Attempt Prediction
Suicide is an important public health problem in America, where it ranks among the ten leading causes of death, with over 14 deaths by suicide per 100,000 persons annually. To solve this pressing problem, a research team at VUMC has created a predictive analytics model. This is a model that uses electronic health records of people to predict the possibility of suicide attempts in particular individuals.
During 11 months at VUMC, the predictive algorithm quietly ran in the background as the doctors focused on their patients. The system was able to predict patients likely to seek health care after committing suicide, thus informing healthcare professionals.
An assistant professor in Biomedical Informatics, Medicine, and Psychiatry, Colin Walsh, highlighted the importance of predictive analytics in healthcare and clinical practice. He observed that even though it is difficult to determine each patient’s suicidal risks for every encounter, the risk model constitutes a vital preliminary screening. This is essential in settings where discussion of suicide risk is not typical, and it also assists in identifying patients that would require further exploration.
8. Improving Patient Engagement
This is important in effective healthcare for active patient involvement. With predictive analytics, the patient’s non-compliance can be detected in advance, and active measures can be taken to keep patients healthy until the next appointment or treatment.
Healthcare providers now make use of predictive analytics in healthcare to design patient profiles that incorporate specially targeted communications and techniques that aid in creating better patient relationships.
Lillian Dittrick, a Fellow of the Society of Actuaries, stresses the need to apply predictive models in identifying and treating responsive patients for lifestyle changes. Predictive analytics is also useful in targeted marketing as it helps to create customer personas based on patient data and customize communication strategies to suit their preferences.
9. Minimizing Missed Appointments
Missed medical appointments and other time-consuming administration efforts cost the US healthcare system about $150 billion a year. Therefore, predictive analytics provides a good way of alerting the hospitals and clinics that have high chances of patients failing in their appointments, thus reducing revenue losses and improving provider satisfaction.
Some researchers at Duke University built a predictive modeling tool that analyzes the EHRs of patients for potential no-shows. The software identified 4,819 cases of no-shows within Duke’s health system. The researchers underscored the need to train the algorithm using local clinical data that produced higher results than vendor training alone.
Community Health Network, in partnership with CipherHealth, a health tech company based in New York, implemented an analytical solution with a view to minimizing cases of patients failing to show up for their appointments and enhancing outreach efforts. The system predicts possible no-shows and also allows for remote consultation adapted to each client.
10. Detecting Early Signs of Sepsis
Sepsis is a deadly condition that the body develops as a result of infection developing quickly. Therefore, predictive analytics can be key in early detection and interventions. Predictive algorithms help in determining patients who are most likely to develop sepsis by continuously monitoring patients’ vital signs and other vital data.
Therefore, for instance, at the University of Pennsylvania Health System, a predictive analytics tool was used to detect potential sepsis patients. The tool used patient data, such as vital signs, lab results, and nursing assessment, in predicting the possibility of sepsis. Through this technology, the hospital was able to decrease early and effective sepsis-related mortality rates
In Conclusion
Predictive analytics is being increasingly used in the healthcare industry, resulting in massive improvements in patient care and operational effectiveness. These real-life applications of predictive analytics in healthcare highlight how powerful predictive analytics can be in transforming the future of predictive analytics in healthcare.
Are you considering incorporating the latest technology into your healthcare services? We being the leader in healthcare software development services, prioritize innovation and client satisfaction. This in turn enables you to provide customizable and scalable healthcare solutions to your patients.
Our proficiency in creating healthcare app solutions is showcased through our various projects such as Soniphi, YouComm, and Health-eApp. We have expertise in delivering innovative platforms powered by artificial intelligence and machine learning in healthcare that give you detailed insights into the behavior and health of your patients so that you can offer better and more streamlined services.
Contact us to understand how you can leverage predictive analytics in your healthcare business.
FAQ’s
Q. How to use predictive analytics in healthcare?
A. Predictive analytics in healthcare utilizes past data to foresee future health incidents and results for early intervention and personalized therapy strategies. This enables it to detect possible health hazards, optimize patient care as well as enhance operations.
Q. What are predictive models used in healthcare?
A. Some of the predictive models commonly applied in healthcare are logistic regression, support vector machines, decision trees, and neural networks. These models analyze patient’s information and predict future effects of these disorders in order to detect diseases prior to their occurrence.
Q. What is an example of predictive analytics in healthcare?
A. One example of predictive analytics in healthcare is the application of machine learning algorithms to determine patient readmissions. This model can analyze historical information and detect patterns of readmissions, allowing healthcare providers to act in time and avoid readmission by patients.
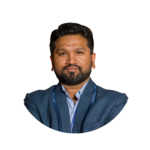
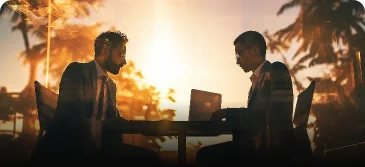
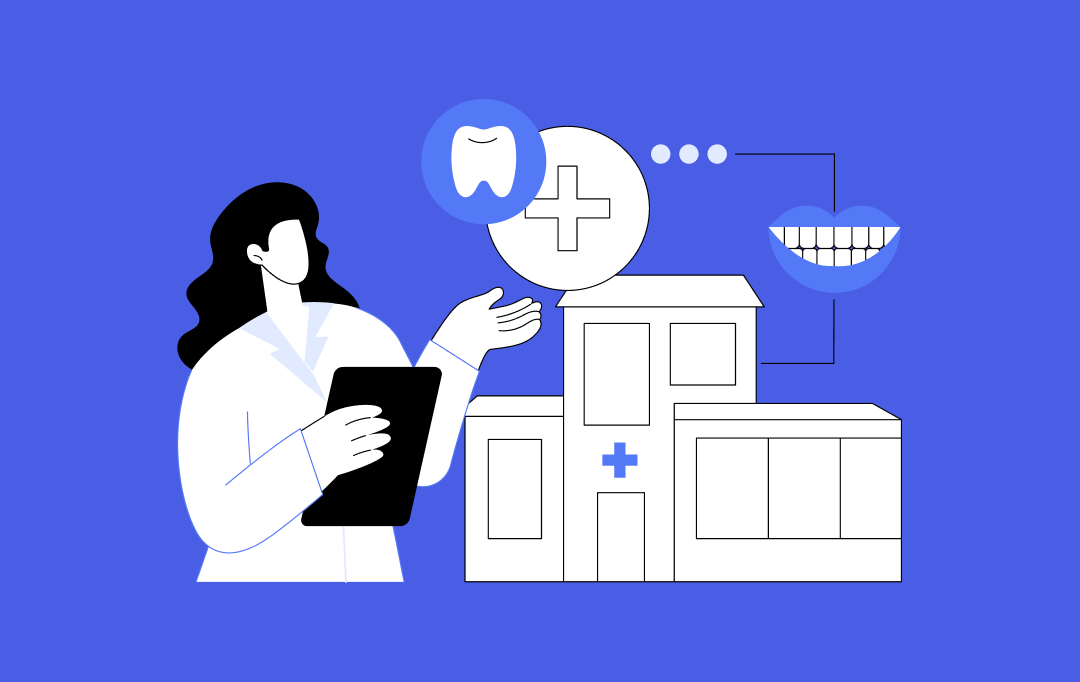
How Much Does it Cost to Build a Custom Dental Practice Management Software?
In recent years, dental practices have shifted away from manual, paper-based systems and embraced advanced software solutions. This change boosts efficiency, enhances patient experiences, and improves data management. While dealing with patient data, dentistry has ditched old-school methods like physical charts, appointment books, and manual billing. They’ve leveled up with a high-tech data handling &…
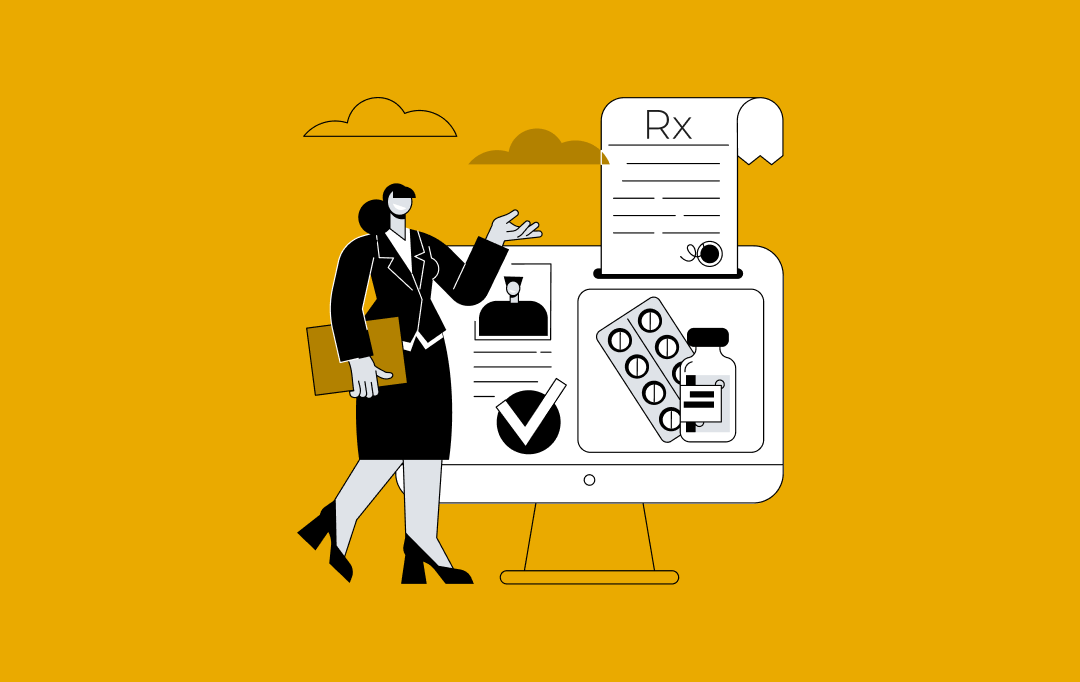
EMR Integration in Healthcare Systems - Benefits, Features, Process, Costs
EMR integration, having proved its worth in the healthcare space with improved workflows, better patient engagements, and lowered operational costs has built itself a projected market share of USD 20.7 billion. Eventhough a range of hospitals swear upon the benefits EMR system integration has garnered them, the adoption level digital healthcare industry anticipated has still…
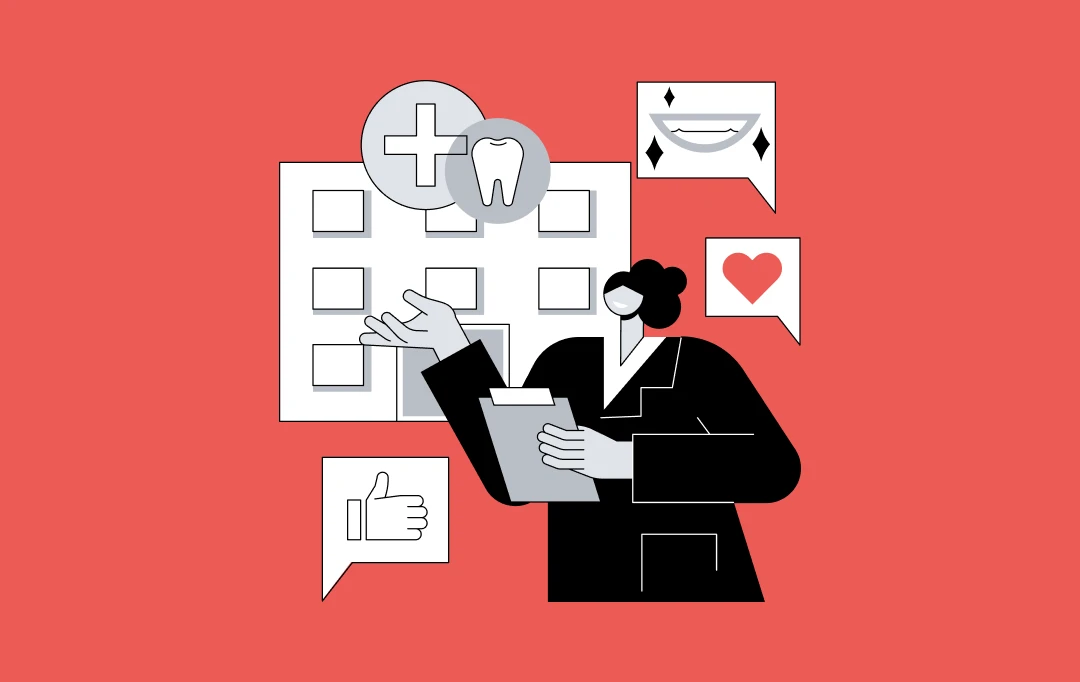