- Understanding the Role of ML in Retail: Key Applications and Advantages
- Demand Prediction and Stock Optimization
- Personalized Recommendations
- Fraud Detection
- Churn Prediction
- Sentiment Analysis
- Dynamic Pricing
- Chatbots and Virtual Shopping Assistants
- Virtual Try-On
- Video Surveillance
- Document Work Automation
- The Future of Machine Learning in Retail
- ML in Retail Challenges and Ways to Overcome Them
- Data Quality and Privacy
- Scalability and Integration
- Talent and Expertise Gap
- How Can Appinventiv Help You Leverage Machine Learning for Retail
- FAQs
In the last few years, the retail industry has faced some critical challenges like the global pandemic — COVID-19, supply chain disruptions, curfews, energy crunches, sustainability pressures, cybersecurity threats, eCommerce apocalypse, and so on.
Despite these obstacles, the industry has shown remarkable resilience, transforming its operations to meet the demands of a rapidly changing retail world. Wondering what is the driving force behind this resilience? It is Artificial Intelligence (AI) and Machine Learning (ML).
Artificial intelligence and machine learning in retail are not just buzzwords; they are the secret sauce, adding flavor to the ever-evolving retail recipe. These advanced tech trends significantly redefine the retail landscape, particularly for companies that count on online sales, where using some kind of AI has become indispensable. It powers everything from personalized shopping experiences to efficient supply chain management.
According to Statista, retailers using these technologies have significantly outperformed their competitors. In both 2023 and 2024, companies adopting AI and machine learning for retail saw double-digit sales growth year-over-year, and their annual profits surged by around 8%, far exceeding those that have not embraced these innovations.
This data highlights the growing acceptance and rapid expansion of AI and machine learning applications in the retail industry. The machine learning use cases in retail are vast and continuously evolving, revolutionizing the industry in ways we couldn’t have imagined a few years back.
This is why many business giants, like Amazon, Alibaba, Walmart, Zara, IKEA, eBay, etc., are already leveraging the benefits of machine learning in retail. These renowned retail companies have implemented ML across their business operations, from product recommendation to inventory management, storage logistics, and post-sale customer service, and reaping significant rewards.
However, you don’t have to be a big corporation to capitalize on the power of machine learning retail solutions. Whether you are selling groceries, apparel, appliances, furniture, or footwear, businesses of all sizes can opt for machine learning applications in retail to unlock immense opportunities.
So, fasten your belt as we take you on a whirlwind tour of how machine learning for retail is redefining the sector. We will explore its key applications, the advantages it offers, the challenges of implementation, and the exciting future potential that lies ahead.
Understanding the Role of ML in Retail: Key Applications and Advantages
The application of machine learning in business addresses several challenges for retailers. With its data analysis, self-learning, and contextual understanding capabilities, machine learning retail solutions help retailers make data-driven decisions, enhancing customer experiences and sales.
To help you gain a better understanding of how ML is changing the retail industry, here are some of the key applications that make it a game-changing solution for retailers:
Demand Prediction and Stock Optimization
Retailers should always ensure that the products in demand are always in stock. Let’s imagine a scenario where a customer plans to host a barbecue, heads to the nearest supermarket to buy some marinated meats, fresh vegetables, and specialty sauces, and finds the shelves empty. Isn’t it a frustrating experience? This stockout situation not only disheartens the customer and leads to a lost sale but also prompts him to seek out a competitor’s store for future purchases. This shift can have long-term consequences, impacting your business revenue.
Demand prediction is a complex task because the buying trends for grocery items and electronic appliances are always different. However, machine learning retail solutions can effectively address this challenge. With ML, retailers can analyze historical sales data, seasonal trends, and consumer behavior to accurately forecast demand. This minimizes overstock and stockout situations while ensuring customers find what they want.
For instance, H&M, a global fashion retailer, uses machine learning in retail for demand prediction and store location optimization. By analyzing customer data through advanced algorithms, H&M accurately forecasts product demand, empowering store managers to effectively manage inventory and maximize store locations. The benefits? Better understanding of customer preferences, increased sales, and more effective resource allocation.
Also Read: How AI in Demand Forecasting Enhancing Supply Chain Efficiency
Personalized Recommendations
Machine learning in the retail industry analyzes vast amounts of customer data to know their demographic details, purchasing preferences, browning history, etc. It helps businesses create tailored offers that resonate with individual shoppers, improving their experience and bond with the brands.
By utilizing ML algorithms, retailers can deliver targeted promotions and personalized offers rather than bombarding every customer with the same sales pitch. Machine learning also empowers retailers to send timely discounts based on customers’ browsing or shopping activity.
For instance, if a customer’s browsing history shows an interest in running shoes, a discount might appear on their “Buy Now” screen right before they consider abandoning their cart before making a final purchase.
Amazon, the retail giant, is a prime example of using machine learning for retail. The company uses ML to analyze customers’ data, recommending relevant items that align perfectly with your interests.
Fraud Detection
A notable application of machine learning in business is fraud detection. How? When it comes to online marketplaces or eCommerce platforms, fraudsters are more likely to steal money from credit cards than from physical products off a shelf. Machine learning plays a vital role in detecting fraudulent activities by analyzing transaction patterns in real-time. By flagging unusual behavior—like a sudden spike in purchases from an unrecognized location—retailers can quickly address potential threats and protect their customers.
FinTech companies that still count on traditional modes of operations often face significant financial losses due to inefficiency in identifying fraudulent activities. With ML in retail, customers feel more protected, knowing that their transactions are in safe hands.
Churn Prediction
Churn prediction is highly effective in monitoring customers’ engagement with products they use daily. By analyzing customer behavior and purchase patterns, machine learning models can effectively identify the potential signs of customer disengagement before they turn into full-blown attrition. By catching these red flags early, retailers can take proactive actions—whether it is sending a personalized email or offering a timely discount—keeping customers glued to the brand and coming back for more.
Sentiment Analysis
Understanding customers’ sentiments about how they feel about your brand or products is more important than ever in today’s digital age. Machine learning applications enable retailers to track customer reviews, social media interactions, and feedback to analyze public sentiment. This insight helps businesses make informed decisions about products and services, ensuring they remain in tune with their audience. After all, a happy customer is the best source of mouth-publicity.
Positive sentiment signals potential opportunities, such as collaborations or product expansions, while negative sentiment acts as an early warning sign for areas needing improvement. This real-time insight allows retailers to adjust their strategies and take appropriate actions.
Dynamic Pricing
Dynamic pricing is one of the most significant machine learning use cases in retail. Gone are the days of fixed prices. With machine learning, retailers can now implement dynamic pricing strategies that adjust product prices in real-time based on customer behavior, competitor prices, and market conditions. These algorithms predict how much a customer is willing to pay for a particular product, enabling retailers to optimize prices for profitability while staying competitive.
For example, machine learning models can scan the web for competitor pricing, hot deals, and promotional activities while also analyzing market trends, product demand, and inventory levels. This data empowers retailers to set smart markdown strategies like discounting outdated products at the end of a season to clear inventory and balance profitability.
Chatbots and Virtual Shopping Assistants
In today’s fast-paced world, customers crave instant gratification and expect immediate responses to their queries. Accordingly, retailers invest in virtual assistants and chatbot development to enhance the customers’ shopping experience.
AI and ML power chatbots and virtual shopping assistants to promptly answer a wide range of queries, provide product recommendations, and guide customers through their shopping journey. These digital helpers are available 24/7, making shopping more convenient and enjoyable.
Virtual Try-On
No more guessing if those running shoes will fit or if that little black dress will suit you! With virtual try-on technology powered by machine learning and augmented reality, customers can see how products will look on them before they actually “buy” them. The benefits? Improved shopping experience, increased customer engagement, reduced return rates, and more confident purchases.
Leading companies like IKEA, MAC Cosmetics, Adidas, Nike, Burberry, Gucci, etc., already use virtual try-on technology to transform the way their customers shop, blurring the lines between offline and online retail.
Video Surveillance
AI and ML-powered video surveillance can help retailers monitor their premises effectively in the age of increasing cyber threats and security concerns. By analyzing footage in real time, these systems can detect suspicious behavior of customers and alert staff immediately. This added layer of security protects assets and enhances the shopping experience.
Document Work Automation
Retail businesses generate heaps of paperwork, from invoices to inventory lists. Using artificial intelligence, NLP, and ML models, retailers can automate document processing, speed up paperwork, and reduce the time spent on mundane tasks. This allows staff to focus on more strategic activities rather than spending their valuable time and effort doing repetitive work.
For instance, manually reviewing 10,000 supplier contracts would typically take around 360,000 hours. However, an intelligent ML model can complete the same task in just a few hours, allowing retailers to streamline supplier management and focus on more strategic tasks.
Also Read: How Can Mobile Apps Add Value to Your Retail Business?
The Future of Machine Learning in Retail
The future of machine learning in retail holds immense potential to revolutionize how businesses operate and interact with customers. As data is a new asset in today’s digital world, we can see that in the coming years, machine learning algorithms will analyze more volume of consumer data in less time, allowing brands to predict customer preferences and tailor recommendations in a jiffy. It will create a more engaging and satisfying shopping experience for buyers, making them feel more valued and privileged.
However, as the reliance on data grows, retailers will need to prioritize ethical considerations, ensuring that their algorithms operate transparently and without bias.
Furthermore, the integration of machine learning with emerging technologies like augmented reality (AR), virtual reality (VR), computer vision, IoT, etc., will unlock new doors for machine learning use cases in retail and redefine the industry.
ML in Retail Challenges and Ways to Overcome Them
While the future and current benefits of machine learning in retail look bright, its implementation comes with its own set of challenges. Let’s delve into some common obstacles retailers often encounter and discuss the strategic solutions for overcoming them.
Data Quality and Privacy
Challenge: Machine learning relies heavily on vast amounts of customer data to deliver accurate insights and personalized experiences. However, ensuring the quality of this data is a key challenge, as incomplete or outdated data can lead to inaccurate forecasts, risking customer privacy. This can result in financial losses, legal penalties, and reputational damage.
Solution: To address the data quality challenge, retailers should adhere to robust data protection and privacy guidelines like GDPR and CCPA. These regulations revolve around three key areas: data collection, data storage, and data usage policy.
Also Read: AI Regulation and Compliance in the US
Scalability and Integration
Challenge: Integrating ML solutions into existing retail systems can be intimidating. Many retailers struggle with integrating legacy systems with advanced ML algorithms, which can lead to inefficiencies and poor system performance.
Solution: To overcome this, retailers should adopt a phased approach, starting with pilot projects and gradually scaling ML applications across the business. Additionally, they can also consider modernizing existing systems to ensure compatibility and smooth integration with new machine learning models.
Talent and Expertise Gap
Challenge: One of the significant challenges retailers face when implementing machine learning is the shortage of skilled talent. Many companies struggle to find qualified tech professionals who can develop, implement, and manage ML models effectively.
Solution: To bridge this gap, businesses should outsource a reputed retail software development company that has proven expertise in machine learning and retail software development.
How Can Appinventiv Help You Leverage Machine Learning for Retail
As we conclude our journey explaining the transformative impact of machine learning in the retail industry, it is evident that ML’s application in business is not just an option but a dire necessity to face evolving business challenges. So, as retailers face new challenges in leveraging state-of-the-art technologies and meeting consumer demands effectively, Appinventiv stands as your trusted tech partner in machine learning retail solutions development and deployment.
As a premium machine learning app development company, we help you navigate the complexities of this innovative technology, transforming your ideas into data-driven solutions that enhance your business outcomes. Whether it is forecasting consumer demands, optimizing inventory management, personalizing customer experiences, or implementing innovative technologies like AR and VR, we stand by you every step of the way.
A testament to our expertise in ML model development is our recent collaboration with a leading wine manufacturer, where we developed an intelligent ML system that predicts wine quality based on its chemical composition. By gathering and analyzing data on various wines, we empower our client to ensure that every bottle meets the highest standards of excellence, aligning with the elite expectations of their discerning customers.
Below is a chart outlining our approach to developing this wine-testing model.
Ready to leverage the full benefits of machine learning in retail and revolutionize your business? Partner with us now and see how we can redefine the future of retail, ensuring that your business not only keeps pace with the modern challenges but also thrives in the digital landscape.
FAQs
Q. How is machine learning used in retail?
A. Depending on the emerging trends of machine learning in retail, the technology can be used in various ways. Here are some of the most practical and profitable use cases of machine learning in retail:
- Demand prediction and stock optimization
- Personalized offer recommendation
- Fraud detection
- Churn prediction
- Sentiment analysis
- Document work automation
- Chatbots and virtual shopping assistants
- Dynamic pricing
- Self-driving vehicles
- Virtual try-on
- Video surveillance
Q. How to implement machine learning in the retail industry?
A. The implementation of ML in retail is a complex task that requires businesses to follow these crucial steps:
- Identify Use Cases: Determine specific challenges or opportunities that you want your ML solution to address.
- Gather Data: Collect and preprocess relevant data from dispersed sources, such as sales transactions, customer interactions, and inventory levels.
- Use Big Data Engineering: Utilize Big Data technologies to manage and process vast amounts of structured and unstructured data to actually see what is going on, what needs to change, and what needs fixing.
- Implement DataOps: Adopt DataOps practices to improve collaboration between DevOps teams and data engineers to streamline data workflows throughout the ML lifecycle.
- Choose Algorithms: Select appropriate machine learning algorithms based on the identified use cases.
- Build and Train ML Models: Develop and train ML models using the gathered data and deploy them at the intended setting for real-time insights.
Q. What is the cost of developing a machine learning model for the retail industry?
A. The cost of developing a machine learning model for retail businesses can vary widely, depending on various factors, including the project’s complexity, the amount and quality of data available, the location and expertise of the ML development company, and so on.
On average, the cost of machine learning model development for retail ranges between $30,000 to $300,000 or more.
To get a more accurate estimate, discuss your project needs with our efficient ML experts and get a detailed quotation tailored to your needs.
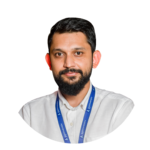
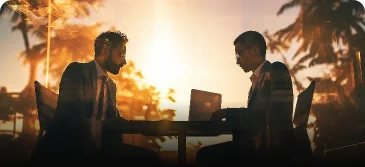
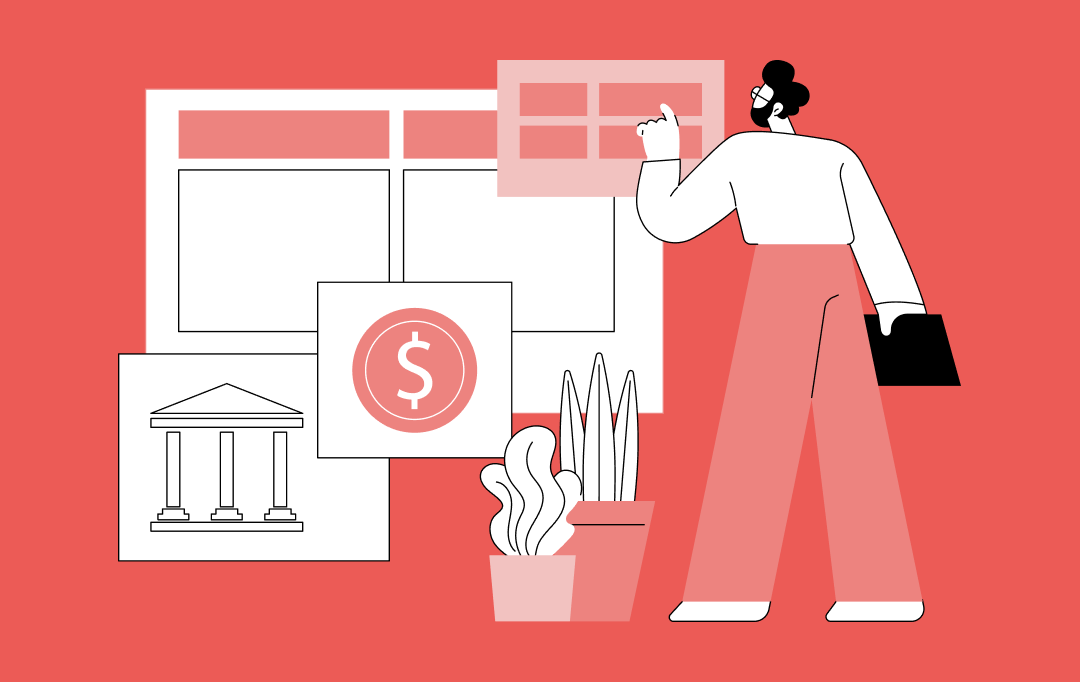
Machine Learning in Banking - Use Cases and Implementation Process
The speed at which fintech transformation is happening is nothing short of a never-seen-before revolution. With the advent of technologies like blockchain, artificial intelligence, metaverse, and edge computing, the fintech space - as we know it - has drastically changed. This transformation's intrinsic change can be seen in how comfortable users have gotten with on-the-move…
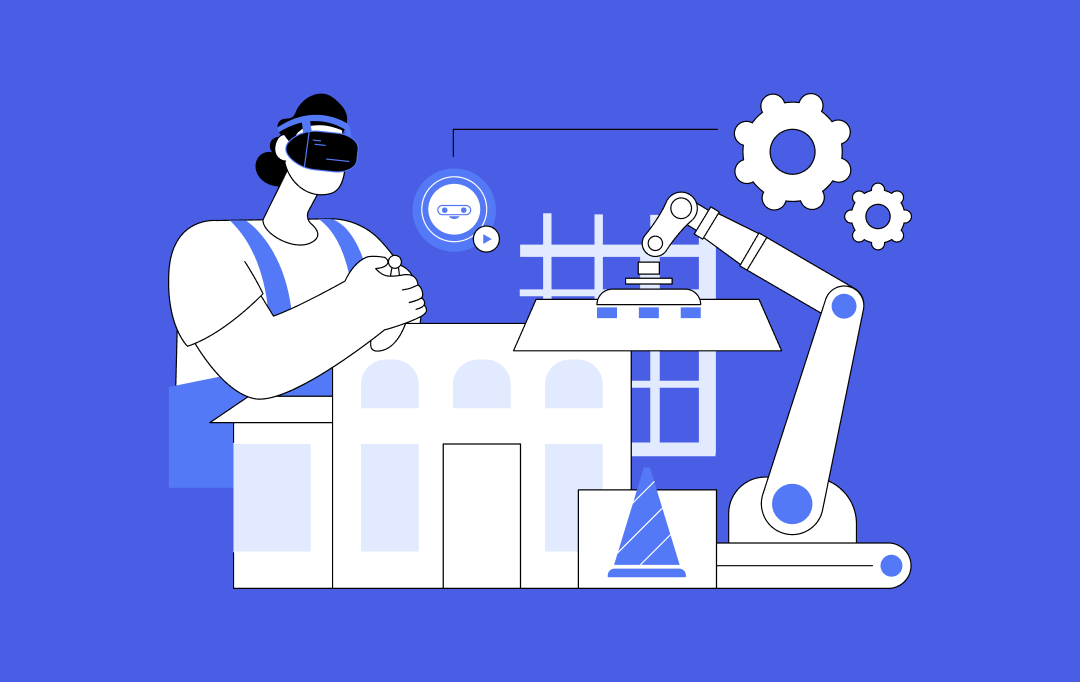
10 Use Cases and Examples of How Machine Learning is Used in Manufacturing
The world is entering the fifth industrial revolution—Industry 5.0—which is giving rise to smart manufacturing that uses artificial intelligence (AI) and machine learning (ML) technologies. Machine learning in manufacturing is not just a buzzword—it is a game-changer. According to MarketsAndMarkets, the Industry 5.0 market is projected to reach $255.7 billion in value by 2029, and…
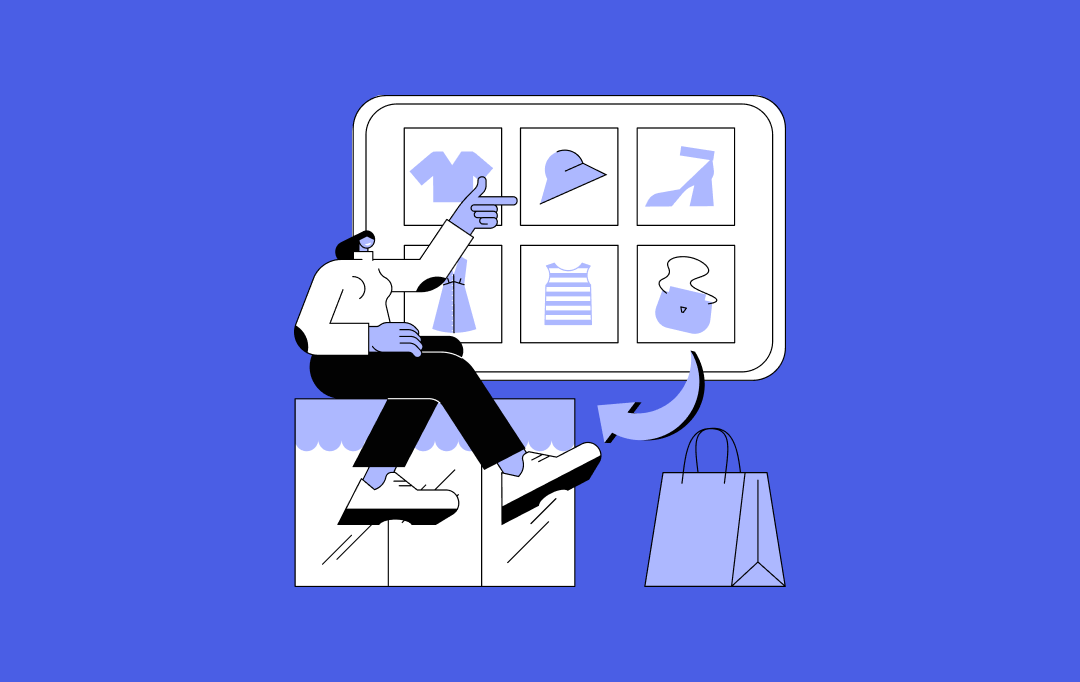