- ML in the Banking Sector: A Profitable Collaboration
- Micro Use Cases of Machine Learning in the Banking Industry
- Back Office Applications of Machine Learning in Banking
- Front Office Applications of ML in Banking Services
- Macro-level Machine Learning Banking Use Cases
- Fraud Detection
- Anti-Money Laundering
- Compliance Regulations
- Customer Retention
- Stock Market Forecasting
- Investment Strategies
- Risk Assessment
- Implementation of Machine Learning Use Cases in Banking
- Step 1: Define Your Business Objectives
- Step 2: Prepare Your Data
- Step 3: Understand Your Algorithms
- Step 4: Foster Employee Adaptation
- How Can Appinventiv Help You Adopt ML for Banking
- FAQs
The speed at which fintech transformation is happening is nothing short of a never-seen-before revolution. With the advent of technologies like blockchain, artificial intelligence, metaverse, and edge computing, the fintech space – as we know it – has drastically changed. This transformation’s intrinsic change can be seen in how comfortable users have gotten with on-the-move transactions, single-click investments, low-effort loan approvals, and the sudden rise in new business models like BNPL and sachet insurance.
While on the customers’ end, tech inclusion has been all about high efficiency and improved lives, traditionally operative banks continue to show signs of struggle. At a time when banks are busy creating and implementing plans for lowering the unbanked population globally, this sudden need for digital transformation comes as an added pressure. However, if market movements are true, this struggle is short-lived, especially with machine learning here to take banks out of this slump.
Let’s dive into it in this article, where we will approach machine learning in banking industry insights in two-fold – use cases and implementation.
ML in the Banking Sector: A Profitable Collaboration
With a market size that is poised to reach $21.27 billion by 2031, machine learning in banking and finance is definitely happening. With use cases being only slightly different from new-gen fintech products, the core functionality remains the same.
Machine Learning in the banking sector works best for pattern identification. They can detect correlations in tons of events and sequences, extracting key information that’s often camouflaged inside massive data sets – something that is easily missed by humans. While seemingly small, this opens doors to a wide range of use cases and applications that have far-reaching outcomes in both micro and macro environment levels.
The ability of ML in the banking sector to learn and predict enables institutions to recognize new business opportunities and work out coherent strategies.
Now that we have a brief overview of how the technology fits into the domain, let us get to the applications part. For this, we will be dividing the use cases of machine learning in banking into two parts – individual banks and the global banking sector as a whole.
Micro Use Cases of Machine Learning in the Banking Industry
In an increasingly digital landscape, machine learning in the banking industry is being applied to enhance both operational efficiency and customer experience. From automating back-office processes to personalizing front-office services, ML technologies are transforming the way financial institutions operate.
In this section, we will explore some key use cases within back-office and front-office functions, highlighting real-world examples that illustrate how banks are successfully implementing these innovative solutions to improve their services and maintain regulatory compliance.
Back Office Applications of Machine Learning in Banking
Let’s delve into specific use cases categorized by essential operational functions within financial institutions, starting with back office.
Document Digitization and Processing
Document digitization refers to the transformation of physical records into digital formats like PDFs or images, facilitating easier storage, access, and management. Technologies such as AI-driven Optical Character Recognition are pivotal in this process, enabling the extraction of text and data from scanned documents. Additionally, Robotic Process Automation can be used to assist in populating data from multiple sources.
On the other end of the coin, there is document processing, which involves the analysis and interpretation of these digitized files to extract pertinent information, verify data accuracy, and take necessary actions based on the document’s content. Algorithms built for implementing machine learning in banking and finance can streamline various document processing tasks, including classification, extraction, validation, and routing.
Example in Practice
Deutsche Bank utilizes AI technologies to streamline manual operations and enhance the classification, extraction, and validation of documents in both back-office functions and customer advisory services. A few of the notable applications include accelerating the traditionally labor-intensive loan document processing using OCR, leveraging AI for portfolio optimization in Wealth Management, and scrutinizing transactions for signs of financial misconduct.
The bank is dedicated to harnessing AI’s capabilities responsibly, ensuring fairness in banking algorithms, and striving for results that are accurate and explainable.
Compliance Monitoring and Reporting
The monitoring of transactions and analysis of data to identify suspicious activities is crucial for compliance in the banking sector, especially for regulations aimed at combating money laundering and terrorist financing. Machine Learning algorithms are instrumental in this use case for automating the identification of suspicious patterns and anomalies in transaction data.
Example in Practice
Standard Chartered Bank employs Machine Learning-driven transaction monitoring systems to bolster its Anti-Money Laundering initiatives and meet regulatory standards. The bank also leverages AI and ML to automate several manual processes and better understand their customer behavior, allowing for an overall more tailored customer experience.
Front Office Applications of ML in Banking Services
Next, let’s explore applications that have a direct impact on customer-facing interactions, products, and services in the banking industry.
Customer Service and Support
According to a recent report, by the end of 2024, 33.2% of adult consumers in the U.S. are expected to engage with AI-enabled banking chatbots. This surge in popularity can be attributed to several advantages chatbots provide, such as:
– Round-the-clock availability
– Immediate responses
– Assistance with transactions
– Continuous learning from user interactions
Example in Practice
Bank of America has made significant strides in the financial sector with Erica, an AI-driven virtual assistant that offers personalized financial advice and support, enhancing customer service and loyalty.
Erica distinguishes itself by utilizing cutting-edge technologies, including advanced analytics and cognitive messaging, to function as a reliable financial advisor. It analyzes various data points from Bank of America, such as a customer’s cash flow, account balances, transaction history, and upcoming bills, helping clients manage their finances effectively.
Personalized Product Recommendations
Machine Learning algorithms assess customer data – ranging from transaction histories to spending behaviors – to create tailored product suggestions and offers. These personalized recommendations are increasingly vital in the banking industry, allowing institutions to cater to the distinct preferences and requirements of individual clients, especially when there are studies that indicate that 78% of banking consumers desire personalized support from their banks.
Example in Practice
Wells Fargo employs Machine Learning engines through its Customer Engagement Engine, which provides relevant product and service recommendations to customers. This solution enhances cross-selling opportunities and boosts customer satisfaction by identifying client needs and delivering what they term ‘next best conversations’ in real-time.
Macro-level Machine Learning Banking Use Cases
As the banking sector evolves, machine learning is becoming a cornerstone of innovation, enhancing operational efficiency and customer experience. From detecting fraud and preventing money laundering to optimizing compliance and personalizing customer service, ML technologies are reshaping the landscape.
This section explores various impactful use cases and machine learning in banking examples highlighting how institutions leverage these tools to address challenges, improve decision-making, and stay competitive in a rapidly changing environment.
Fraud Detection
As cybercriminals continuously evolve their tactics, banks must enhance their defenses against financial crimes such as fraud and money laundering. Implementing fraud detection in banking using machine learning enables banks to identify malicious activities swiftly, verify user identities, and respond effectively to cyber threats.
ML algorithms can also process vast amounts of transaction data in real time, significantly reducing the need for human intervention. These systems are built to quickly recognize suspicious activities, alerting security teams or automatically blocking fraudulent transactions.
Notable startups in this space of banking machine learning use cases include:
- Resistant.ai: Focuses on protecting AI systems from adversarial attacks and fraud.
- Riskified: Utilizes ML to provide insights that help businesses decide on transaction approvals.
- Feedzai: Develops risk management tools aimed at preventing fraud and money laundering.
Anti-Money Laundering
Machine learning plays a pivotal role in enhancing Anti-Money Laundering initiatives by analyzing extensive financial data to identify patterns indicative of illicit activities.
The algorithms can automatically detect unusual transaction behavior, flagging it for further investigation by learning users normal transaction patterns and identifying anomalies such as large or rapid transactions and activity directed towards high-risk jurisdictions. Additionally, they can unveil complex money laundering schemes, including layering techniques and smurfing, which obscure the origins of funds.
Banks can also apply the tech to create dynamic risk profiles by assessing customer data and transaction history, helping identify high-risk clients and allocate resources effectively.
One example of an innovative ML application for AML is Google Cloud’s Anti-Money Laundering AI, which generates customer risk scores based on transactional and KYC data.
Compliance Regulations
Financial institutions worldwide face constant pressure to adhere to changing regulations, often leading to operational disruptions and potential fines. Machine learning offers a solution to this by processing regulatory documents and detecting correlations between guidelines, enabling institutions to respond promptly to legal changes.
Startups working for this lot of machine learning banking use cases include:
- Zest.ai: Aids lenders in making better decisions and automating compliance.
- ComplyAdvantage: Provides AI-driven financial crime risk data to assist in regulatory compliance.
- Sym: Bridges the gap between policies and workflows to ensure compliance.
[Also Read: How to develop a PCI-compliant application?]
Customer Retention
With financial institutions inundated with support requests, machine learning can streamline and enhance customer service processes. By analyzing vast datasets, ML enables personalized assistance and targeted offers, improving customer experiences. Chatbots are the tools which tend to exemplify this application of machine learning in the banking sector, providing real-time, human-like support that evolves with each interaction.
The technology ultimately reduces the need for extensive customer support departments, benefiting both large and small financial institutions.
Prominent live examples of retention-specific machine learning banking use cases include:
- Wells Fargo: Introduced an ML-driven assistant for Facebook Messenger.
- Bank of America: Launched Erica, an ML-powered virtual assistant that saw rapid user growth during the pandemic.
Stock Market Forecasting
Another of the key benefits of machine learning in banking can be seen in the tech offering powerful tools for predicting stock market dynamics by analyzing extensive historical datasets alongside real-time data. ML algorithms can also forecast stock prices and market trends, aiding traders in developing algorithmic trading systems.
Examples of ML applications for stock predictions include:
- Walnut Algorithms: Provides AI and ML solutions for investment management.
- QARA: Utilizes deep learning to analyze financial markets through a mobile application.
[Also Read: How AI is transforming the stock trading market]
Investment Strategies
Machine learning is reshaping investment strategies by uncovering patterns in historical and real-time financial data. This data-driven approach engines predictive analytics, which provides investors deeper insights into market movements.
Additionally, in algorithmic trading, ML automates trades based on learned strategies, allowing for rapid adaptation to market changes. Lastly, it also aids in portfolio optimization by adjusting asset allocations based on risk tolerance and market conditions.
Companies utilizing ML for investment strategies include:
- BlackRock: Employs ML in its Aladdin Wealth platform to tailor investment portfolios.
- Trade Ideas: Offers an AI-powered robo-advisor that analyzes trading scenarios to identify optimal investment opportunities.
Risk Assessment
Machine learning has the potential to significantly enhance risk assessment processes in banking, particularly in credit scoring. By analyzing diverse data sources, like rent payments and telecommunications data, ML algorithms can provide a comprehensive risk profile for loan applicants in a matter of a few minutes.
Notable examples of companies exploring machine learning in banking industry for credit scoring include:
- Kreditech: Assists in evaluating the creditworthiness of potential borrowers without extensive banking histories.
- Aire: Offers credit assessment services that provide new credit scores for better access to financial products.
With the use cases of machine learning for banks now explored, it goes without saying that the success would depend entirely on how the technology gets implemented in the banking domain. Let’s look into that in the next section.
Implementation of Machine Learning Use Cases in Banking
Successfully integrating machine learning into banking operations requires a strategic approach that aligns with your organization’s goals. The steps we will be talking about can help facilitate the implementation process, from identifying specific business cases to preparing your data and understanding the algorithms at play.
Each step of the machine learning for banks implementation emphasizes on the importance of collaboration and communication across the organization, ensuring that employees are equipped to adapt to this transformative technology. By following this, or similar, structured roadmap, banks can effectively leverage machine learning to enhance operations, improve customer experiences, and drive innovation.
Step 1: Define Your Business Objectives
Before diving into machine learning implementation, bank leaders should start by clearly defining their strategic goals. This understanding of how ML can support these objectives is crucial for not just maintaining clear communication of the vision throughout the organization but also for managers to identify specific ML opportunities within their divisions.
No matter what the vision is, it’s advisable to focus on quick wins – easy-to-implement solutions that demonstrate fast returns on investment. This approach can help uncover technological challenges and facilitates the development of a long-term ML strategy.
Step 2: Prepare Your Data
The effectiveness of machine learning is heavily dependent on the quality of the data used. Banks, at all times, should prioritize data accuracy and compliance with relevant regulations to prevent issues such as data breaches, which can severely damage customer trust.
To ensure high data quality, banking institutes can adopt the following practices:
- Data Cleansing at Capture: Establish processes to clean data as it is entered.
- Proper Data Labeling: Ensure that data is appropriately labeled for supervised learning.
- Unique Identification Systems: Implement a unique numbering system to facilitate data cross-referencing across multiple databases.
- Maintain a “Golden Copy”: Keep a cleansed, consolidated version of data from various sources for compliance and analysis.
- Regular Updates: Update data frequently to mitigate decay, which can occur at an annual rate of 25% in financial institutions.
Step 3: Understand Your Algorithms
It’s essential for banks to familiarize themselves with various machine learning algorithms and their implications. In our experience, understanding how different algorithms function, including their strengths and weaknesses, can significantly influence decision-making when looking at the risk-reward of the use cases of machine learning in banking.
- Decision Trees: Useful for classification and regression tasks, decision trees provide clear, interpretable models that can help in credit scoring and risk assessment.
- Random Forest: An ensemble method that builds multiple decision trees and combines their outputs, random forests improve accuracy and reduce overfitting, making them ideal for fraud detection.
- Gradient Boosting Machines: This technique builds models in a stage-wise fashion and is effective for handling various types of predictive modeling tasks, such as customer churn prediction.
- Support Vector Machines: Useful for classification problems, SVMs can help distinguish between different classes of transactions, such as legitimate vs. fraudulent ones.
- Neural Networks: Particularly useful for complex tasks, deep learning models can analyze large datasets for various applications, including natural language processing for chatbots.
- k-Nearest Neighbors: A simple yet effective algorithm for classification and regression tasks, k-NN can be used in customer segmentation and credit scoring.
- Naive Bayes: This algorithm is effective for classification problems and is often used in spam detection and customer sentiment analysis.
- Anomaly Detection Algorithms: Techniques such as Isolation Forest and One-Class SVM are specifically designed to identify outliers, making them valuable for fraud detection and monitoring suspicious transactions.
Step 4: Foster Employee Adaptation
The introduction of machine learning in the banking industry can create uncertainty among employees, leading to concerns about job security or skepticism regarding the initiative’s longevity. To ensure a smooth transition, organizations must create a clear vision and roadmap for integrating ML into daily operations.
Some of the key strategies to smoothen out the process can include:
- Transparent Communication: Clearly articulate the goals and timelines of the ML initiative across all levels of the organization.
- Cultural Shift: Encourage a collaborative environment that embraces change. Employees should understand how ML can enhance their roles by taking over repetitive tasks and allowing them to focus on more creative and strategic activities.
To see this through, employees will need to adapt to new roles as the facilitators of machine learning:
- Trainers: Help algorithms learn from data effectively.
- Explainers: Interpret and communicate the results of algorithms, ensuring compliance with regulations.
- Sustainers: Monitor algorithms to prevent bias and ensure they evolve in line with customer behavior.
If there’s anything (or two things) that we would like to present as a summary here, they are – A. The use cases or benefits of machine learning in banking are too big to ignore. B. The implementation of machine learning in the banking sector is easier read than done.
This is where Appinventiv, as a banking software development company, comes into the picture.
How Can Appinventiv Help You Adopt ML for Banking
With an experience of working with multiple US and Middle East regional banks on their journey towards the exploration of the impact of machine learning in banking, we have an in-depth experience into not just helping banks transform digitally but also scaling up with the inclusion of next-gen technologies like AI-ML, Blockchain, Advanced Computing implementation, etc.
Our team of machine learning services providers have all the necessary skill sets to build a software or process that helps banks come at par with the digital movements of the current world. The result can be seen through a series of laudable stats ranging from a 60% reduction in fraudulent transactions to a 50% decrease in loan approval times, showcasing our impact on efficiency, security, and customer satisfaction in the banking sector.
Looking for similar results? Get in touch with the banking software development company that the world trusts, today.
FAQs
Q. What is machine learning in banking?
A. Machine learning in the banking industry refers to the use of algorithms and statistical models to analyze and interpret complex data sets, enabling financial institutions to make data-driven decisions.
By leveraging historical data, machine learning can identify patterns, predict outcomes, and automate processes, thereby enhancing efficiency, reducing risks, and improving customer experiences. The application of this can be seen in areas such as credit scoring, fraud detection, customer service, and regulatory compliance.
Q. How can machine learning be used in banking?
A. The use cases of machine learning in banking can be seen along several impactful ways, including:
- Fraud Detection: ML algorithms analyze transaction patterns in real-time to identify and flag suspicious activities, reducing the risk of financial crime.
- Customer Service: AI-powered chatbots can provide personalized assistance and support, improving customer engagement and satisfaction.
- Risk Management: Machine learning enhances risk assessment processes by analyzing various data sources to effectively predict potential defaults and losses.
- Regulatory Compliance: ML systems can monitor regulatory changes and automate compliance processes, helping banks adhere to evolving laws and standards more efficiently.
- Personalized Marketing: By analyzing customer behavior and preferences, banks can tailor their products and services to meet individual needs, ultimately enhancing their customers’ loyalty.
Q. What can be one prime example of machine learning in banking?
A. A notable example of machine learning in the banking sector is the use of predictive analytics for credit scoring. Financial institutions can analyze a wide range of data points, including transaction history, social media activity, and even behavioral patterns, to assess a borrower’s creditworthiness.
A real world example of this set of machine learning use cases in banking can be seen through ZestFinance, which utilizes machine learning algorithms to create more accurate credit models, enabling them to approve loans for individuals who may have been overlooked by traditional scoring methods.
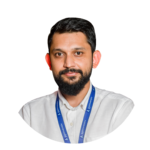
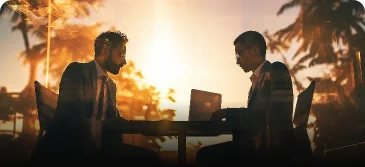
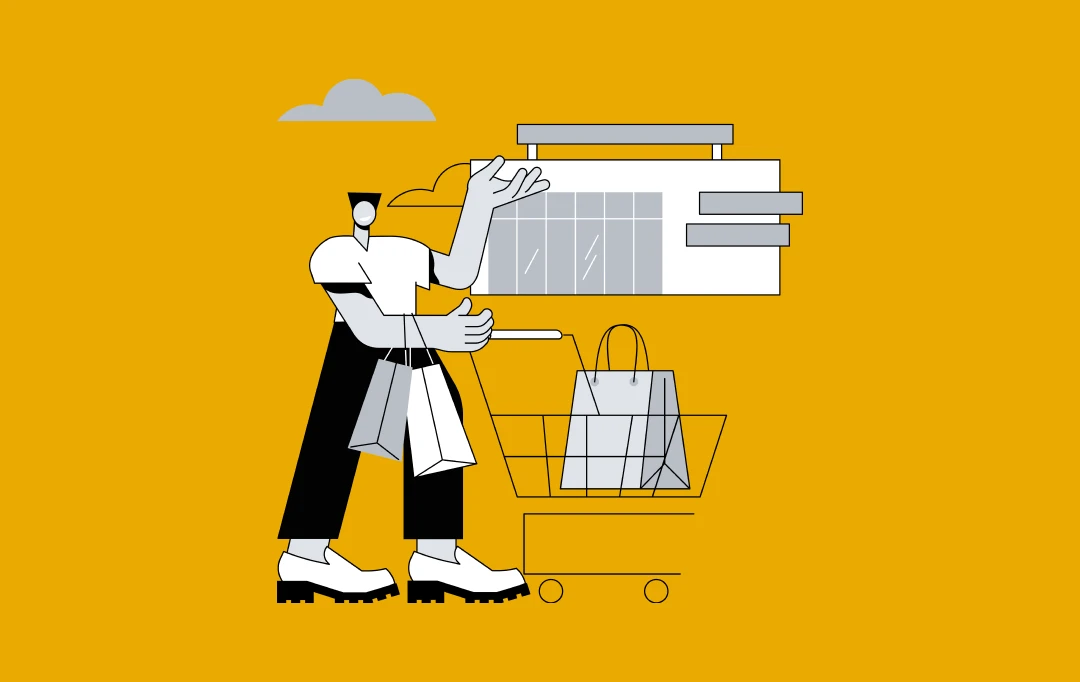
How Machine Learning in Retail is Redefining the Sector - Key Business Opportunities and Challenge
In the last few years, the retail industry has faced some critical challenges like the global pandemic — COVID-19, supply chain disruptions, curfews, energy crunches, sustainability pressures, cybersecurity threats, eCommerce apocalypse, and so on. Despite these obstacles, the industry has shown remarkable resilience, transforming its operations to meet the demands of a rapidly changing retail…
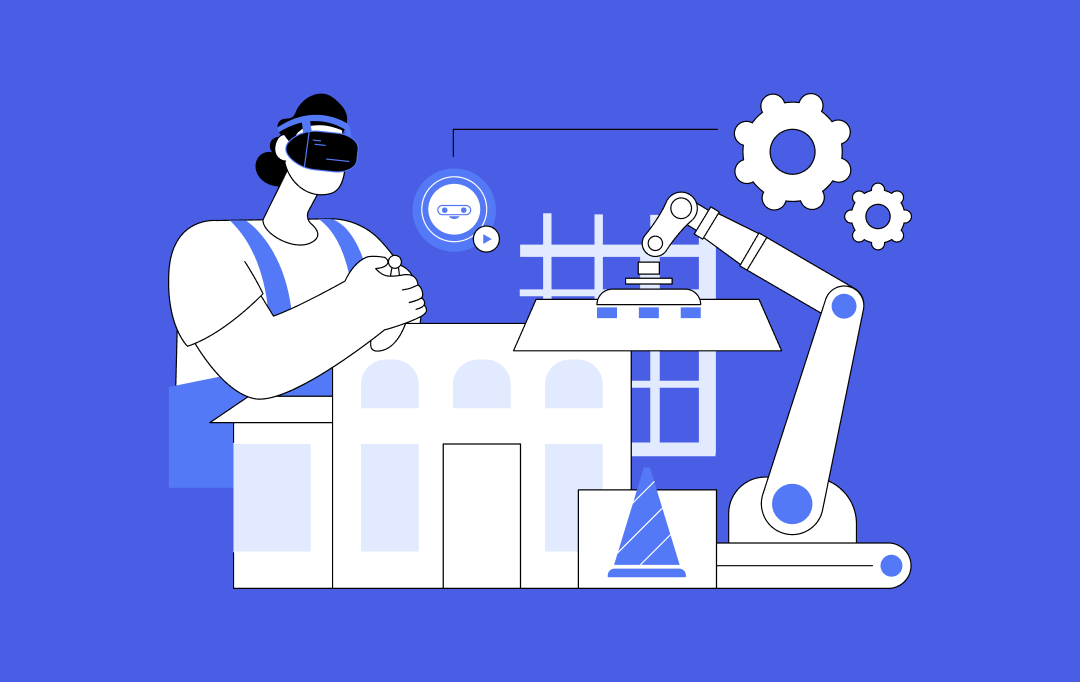
10 Use Cases and Examples of How Machine Learning is Used in Manufacturing
The world is entering the fifth industrial revolution—Industry 5.0—which is giving rise to smart manufacturing that uses artificial intelligence (AI) and machine learning (ML) technologies. Machine learning in manufacturing is not just a buzzword—it is a game-changer. According to MarketsAndMarkets, the Industry 5.0 market is projected to reach $255.7 billion in value by 2029, and…
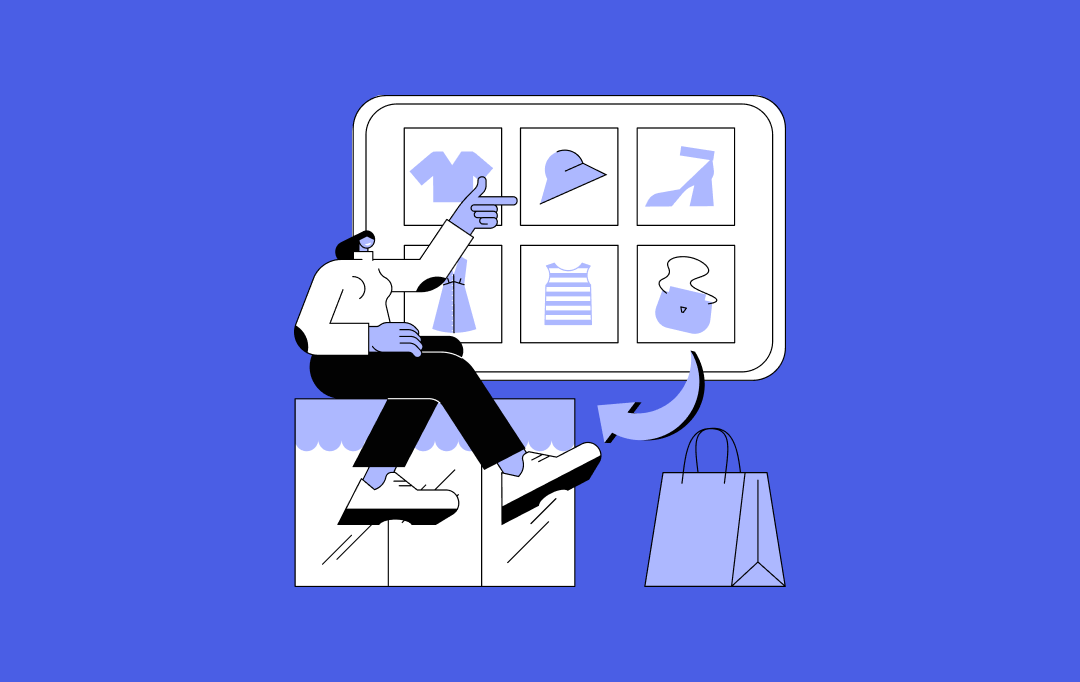